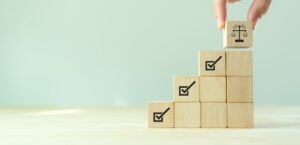
(3rdtimeluckystudio/Shutterstock)
Monetary providers companies are desirous to undertake generative AI to chop prices, develop revenues, and enhance buyer satisfaction, as are many organizations. Nevertheless, the dangers related to GenAI aren’t trivial, particularly in monetary providers. TCI Media not too long ago hosted specialists from EY to assist practitioners in FinServ get began on their accountable AI journeys.
In the course of the latest HPC + AI on Wall Avenue occasion, which passed off in New York Metropolis in mid-September, TCI Media welcomed two AI specialists from EY Americas, together with Rani Bhuva, a principal at EY and EY Americas Monetary Providers Accountable AI Chief, and Kiranjot Dhillon, a senior supervisor at EY and the EY Americas Monetary Providers AI Chief.
Of their speak, titled “Accountable AI: Regulatory Compliance Traits and Key Concerns for AI Practitioners,” Bhuva and Dhillon mentioned lots of the challenges that monetary providers companies face when making an attempt to undertake AI, together with GenAI, in addition to a few of the steps that firms can take to get began.
FinServ firms are not any stranger to regulation, they usually’ll discover loads of that taking place in GenAI all over the world. The European Union’s AI Act has gained a number of consideration globally, whereas right here within the US, there are about 200 potential knowledge and AI payments being drawn up on the state stage, in line with Bhuva.
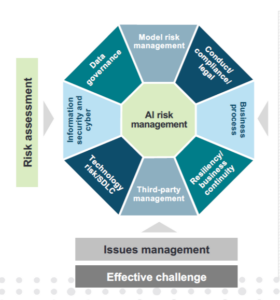
Firms should carry numerous specialists to the desk to develop and deploy AI responsibly (Picture courtesy EY)
On the federal stage, many of the motion to this point has occurred with the NIST AI Framework from early 2023 and President Joe Biden’s October 2023 govt order, she stated. The Federal Communications Fee (FCC) and the Federal Reserve have instructed monetary companies to take the rules they’ve handed over the previous 10 years round mannequin threat administration and governance, and apply that to GenAI, she stated.
Federal regulators are nonetheless principally in studying mode on the subject of GenAI, she stated. Many of those businesses, similar to US Treasury and Client Monetary Safety Board (CFPB), have issued requests for data (RFIs) to get suggestions from the sector, whereas others, just like the Monetary Business Regulatory Authority (FINR) and the Securities and Trade Fee (SEC) have clarified some rules round buyer knowledge in AI apps.
“One factor that has clearly emerged is alignment with the NIST,” Bhuva stated. “The problem from the NIST, in fact, is that it hasn’t taken under consideration the entire different regulatory issuances inside monetary providers. So if you concentrate on what’s occurred up to now decade, mannequin threat administration, TPRM [third-party risk management], cybersecurity–there’s much more element inside monetary providers regulation that applies in the present day.”
So how do FinServ firms get began? EY recommends that firms take a step again and take a look at the instruments they have already got in place. To make sure compliance, firms ought to take a look at three particular areas.
- AI governance framework – An overarching framework that encompasses mannequin threat administration, TPRM [third-party risk management], and cybersecurity;
- AI stock – A doc describing the entire AI elements and belongings, together with machine studying fashions and coaching knowledge, that your organization has developed to date;
- AI reporting – A system to watch and report on the performance of AI methods, particularly high-risk AI methods.
GenAI is altering rapidly, and so are the dangers that it entails. The NIST not too long ago issued steering on how to deal with GenAI-specific dangers. EY’s Bhuva known as out one of many dangers: the tendency for AI fashions to make issues up.
“Everybody’s been utilizing the time period ‘hallucination,’ however the NIST is particularly involved with anthropomorphization of AI,” she stated. “And so the thought was that hallucination makes AI appear too human. So that they got here up with the phrase confabulation to explain that. I haven’t truly heard anybody use confabulation. I believe everyone seems to be caught on hallucinations, however that’s on the market.”
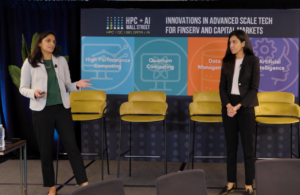
Rani Bhuva (left), a principal at EY and EY Americas Monetary Providers Accountable AI Chief, and Kiranjot Dhillon, a senior supervisor at EY and the EY Americas Monetary Providers AI Chief, current at 2024 HPC + AI on Wall Avenue
There are a number of features to accountable AI improvement, and bringing all of them collectively right into a cohesive program with efficient controls and validation just isn’t a simple process. To do it proper, an organization should adapt quite a lot of separate applications, the whole lot from mannequin threat administration and regulatory compliance to knowledge safety and enterprise continuity. Simply getting everybody to work collectively in the direction of this finish is a problem, Bhuva stated.
“You actually need to make it possible for the suitable events are on the desk,” she stated. “This will get to be pretty difficult as a result of it’s good to have completely different areas of experience, given the complexity of generative AI. Are you able to discuss whether or not or not you’ve successfully addressed all controls related to generative AI except you examine the field on privateness, except you examine the field on TPRM, knowledge governance, mannequin threat administration as effectively?”
Following rules of moral AI is one other ball of wax altogether. For the reason that guidelines of moral AI usually aren’t legally enforceable, it’s as much as a person firm whether or not they’ll keep away from utilizing massive language fashions (LLMs) which were educated on copyrighted knowledge, Bhuva identified. And even when an LLM supplier indemnifies your organization towards copyright lawsuits, is it nonetheless moral to make use of the LLM if you recognize it was educated on copyrighted knowledge anyway?
“One other problem, if you concentrate on the entire assurances on the world stage, all of them discuss privateness, they discuss equity, they discuss explainability, accuracy, safety–the entire rules,” Bhuva stated. “However a number of these rules battle with one another. So to make sure that your mannequin is correct, you essentially want a number of knowledge, however you is likely to be violating potential privateness necessities with a purpose to get all that knowledge. You is likely to be sacrificing explainability for accuracy as effectively. So there’s loads you could’t resolve for from a regulatory or legislative perspective, and in order that’s why we see a number of curiosity in AI ethics.”
Implementing Accountable GenAI
EY’s Kiranjot Dhillon, who’s an utilized AI scientist, supplied a practitioner’s view of accountable AI to the HPC + AI on Wall Avenue viewers.
One of many massive challenges that GenAI practitioners are dealing with proper now–and one of many massive the explanation why many GenAI apps haven’t been put into manufacturing–is the problem in realizing precisely how GenAI methods will truly behave in operational environments, Dhillon stated.
“One of many key root causes is pondering by accountable AI and these threat mitigation practices in the direction of the top of the solution-build life cycle,” she stated. “It’s extra retrofitting and overlaying these necessities to make it possible for they’re met, versus desirous about that every one the best way on the onset.”
It’s exhausting to get readability into that whenever you construct the instrumentation that can inform that query into the system after-the-fact. It’s significantly better to do it from the start, she stated.
“Accountable necessities have to be thought by proper on the initialization stage,” Dhillon stated. “And applicable toll gates have to be then trickled by the person subsequent steps and go right through operationalization and acquiring approvals in these particular person steps as effectively.”
Because the bones of the GenAI methods are laid down, it’s vital for the builders and designers to consider the metrics and different standards they need to accumulate to make sure that they’re assembly their accountable AI targets, she stated. Because the system, it’s as much as the accountable AI crew members–and probably even a problem crew, or a “pink crew”–to step in and choose whether or not the necessities truly are being met.
Dhillon helps using person guardrails to construct accountable GenAI methods. These methods, similar to Nvidia’s Nemo, can work on each the enter to the LLM in addition to the output. They will stop sure requests from reaching the LLM, and instruct the LLM to not reply in sure methods.
“You possibly can take into consideration topical guardrails the place the answer just isn’t digressing from the subject at hand,” Dhillon stated. “It may very well be security and safety guardrails, like making an attempt to scale back hallucinations or confabulations, after we speak within the NIST phrases, and making an attempt to floor the answer increasingly more. Or stopping the answer from reaching out to an exterior, doubtlessly unsafe purposes proper on the supply stage. So having these eventualities recognized, captured, and tackled is what guardrails actually present us.”
Typically, the most effective guardrail for an LLM is a second LLM that can oversee the primary LLM and maintain a watch out for issues like logical correctness, reply relevancy, or context relevancy, that are very talked-about within the RAG area, Dhillon stated.
There are additionally some finest practices on the subject of utilizing immediate engineering in a accountable method. Whereas the information is relied upon to coach the mannequin in conventional machine studying, in GenAI, it’s generally finest to provide the pc express directions to observe, Dhillon stated.
“It’s pondering by what you’re on the lookout for the LLM to do and instructing it precisely what you count on for it to do,” she stated. “And it’s actually so simple as that. Be descriptive. Be verbose in what your expectations of the LLM and instructing it to not reply when sure queries are requested, or answered on this approach or that approach.”
Relying on which kind of prompting you utilize, similar to zero-shot prompting, few-shot prompting, or chain-of-thought prompting, the robustness of the ultimate GenAI resolution will differ.
Lastly, it’s essential to have a human within the loop to watch the GenAI system and be sure that it’s not going off the rails. Throughout her presentation, Dhillon confirmed how having a visualization software that may use automated statistical methods to cluster numerous responses will help the human rapidly spot any anomalies or outliers.
“The thought right here is {that a} human evaluator may rapidly take a look at it and see that stuff that’s falling inside that larger, bean formed cluster could be very near what the information base is, so it’s most definitely related queries,” she stated. “And as you begin to sort of go additional away from that key cluster, you’re seeing queries which may perhaps be tangentially associated. All the best way to the underside left is a cluster which is, upon handbook evaluate, turned out to be profane queries. In order that’s why it’s very far off within the visualized dimensional area from the stuff that you’d count on the LLM to be answered. And at that time, you’ll be able to create the proper sorts of triggers to usher in handbook intervention.”
You may watch Bhava and Dhillon’s full presentation, in addition to the opposite recorded HPC +
AI on Wall Avenue shows, by registering at www.hpcaiwallstreet.com.
Associated Gadgets:
Biden’s Government Order on AI and Knowledge Privateness Will get Largely Favorable Reactions
Bridging Intent with Motion: The Moral Journey of AI Democratization
NIST Places AI Danger Administration on the Map with New Framework