As AI evolves, efficient collaboration throughout venture lifecycles stays a urgent problem for AI groups.
The truth is, 20% of AI leaders cite collaboration as their largest unmet want, underscoring that constructing cohesive AI groups is simply as important as constructing the AI itself.
With AI initiatives rising in complexity and scale, organizations that foster robust, cross-functional partnerships achieve a essential edge within the race for innovation.
This fast information equips AI leaders with sensible methods to strengthen collaboration throughout groups, guaranteeing smoother workflows, quicker progress, and extra profitable AI outcomes.
Teamwork hurdles AI leaders are dealing with
AI collaboration is strained by crew silos, shifting work environments, misaligned aims, and rising enterprise calls for.
For AI groups, these challenges manifest in 4 key areas:
- Fragmentation: Disjointed instruments, workflows, and processes make it tough for groups to function as a cohesive unit.
- Coordination complexity: Aligning cross-functional groups on hand-off priorities, timelines, and dependencies turns into exponentially more durable as initiatives scale.
- Inconsistent communication: Gaps in communication result in missed alternatives, redundancies, rework, and confusion over venture standing and tasks.
- Mannequin integrity: Making certain mannequin accuracy, equity, and safety requires seamless handoffs and fixed oversight, however disconnected groups typically lack the shared accountability or the observability instruments wanted to keep up it.
Addressing these hurdles is essential for AI leaders who wish to streamline operations, decrease dangers, and drive significant outcomes quicker.
Fragmentation workflows, instruments, and languages
An AI venture sometimes passes by 5 groups, seven instruments, and 12 programming languages earlier than reaching its enterprise customers — and that’s only the start.
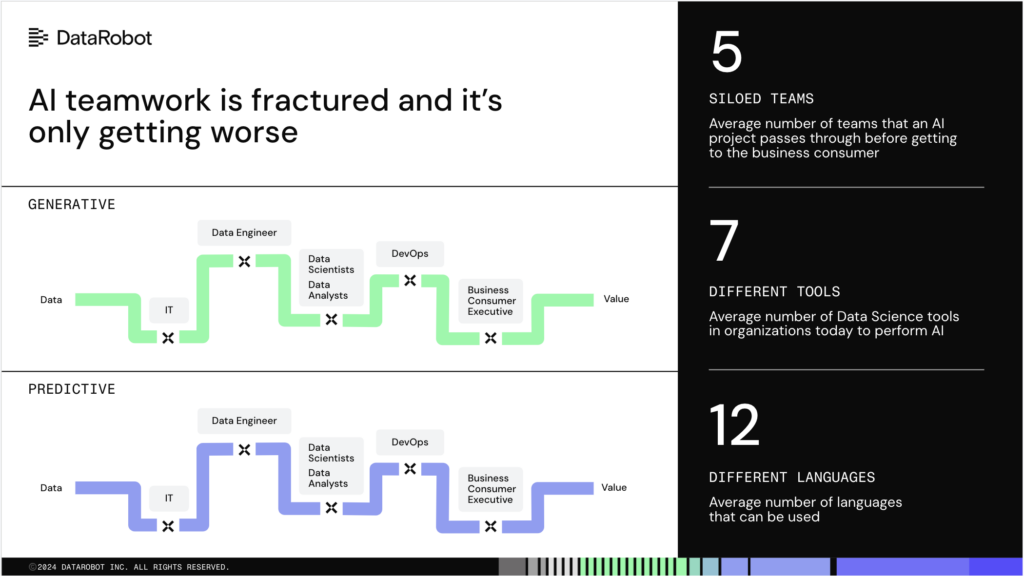
Right here’s how fragmentation disrupts collaboration and what AI leaders can do to repair it:
- Disjointed initiatives: Silos between groups create misalignment. Throughout the starting stage, design clear workflows and shared targets.
- Duplicated efforts: Redundant work slows progress and creates waste. Use shared documentation and centralized venture instruments to keep away from overlap.
- Delays in completion: Poor handoffs create bottlenecks. Implement structured handoff processes and align timelines to maintain initiatives transferring.
- Device and coding language incompatibility: Incompatible instruments hinder interoperability. Standardize instruments and programming languages the place attainable to boost compatibility and streamline collaboration.
When the processes and groups are fragmented, it’s more durable to keep up a united imaginative and prescient for the venture. Over time, these misalignments can erode the enterprise impression and consumer engagement of the ultimate AI output.
The hidden value of hand-offs
Every stage of an AI venture presents a brand new hand-off – and with it, new dangers to progress and efficiency. Right here’s the place issues typically go flawed:
- Knowledge gaps from analysis to growth: Incomplete or inconsistent information transfers and information duplication sluggish growth and will increase rework.
- Misaligned expectations: Unclear testing standards result in defects and delays throughout development-to-testing handoffs.
- Integration points: Variations in technical environments could cause failures when fashions are moved from check to manufacturing.
- Weak monitoring: Restricted oversight after deployment permits undetected points to hurt mannequin efficiency and jeopardize enterprise operations.
To mitigate these dangers, AI leaders ought to provide options that synchronize cross-functional groups at every stage of growth to protect venture momentum and guarantee a extra predictable, managed path to deployment.
Strategic options
Breaking down obstacles in crew communications
AI leaders face a rising impediment in uniting code-first and low-code groups whereas streamlining workflows to enhance effectivity. This disconnect is critical, with 13% of AI leaders citing collaboration points between groups as a serious barrier when advancing AI use circumstances by numerous lifecycle levels.
To deal with these challenges, AI leaders can give attention to two core methods:
1. Present context to align groups
AI leaders play a essential position in guaranteeing their groups perceive the total venture context, together with the use case, enterprise relevance, meant outcomes, and organizational insurance policies.
Integrating these insights into approval workflows and automatic guardrails maintains readability on roles and tasks, protects delicate information like personally identifiable info (PII), and ensures compliance with insurance policies.
By prioritizing clear communication and embedding context into workflows, leaders create an setting the place groups can confidently innovate with out risking delicate info or operational integrity.
2. Use centralized platforms for collaboration
AI groups want a centralized communication platform to collaborate throughout mannequin growth, testing, and deployment levels.
An built-in AI suite can streamline workflows by permitting groups to tag property, add feedback, and share assets by central registries and use case hubs.
Key options like automated versioning and complete documentation guarantee work integrity whereas offering a transparent historic document, simplify handoffs, and maintain initiatives on monitor.
By combining clear context-setting with centralized instruments, AI leaders can bridge crew communication gaps, remove redundancies, and keep effectivity throughout all the AI lifecycle.
Defending mannequin integrity from growth to deployment
For a lot of organizations, fashions take greater than seven months to succeed in manufacturing – no matter AI maturity. This prolonged timeline introduces extra alternatives for errors, inconsistencies, and misaligned targets.
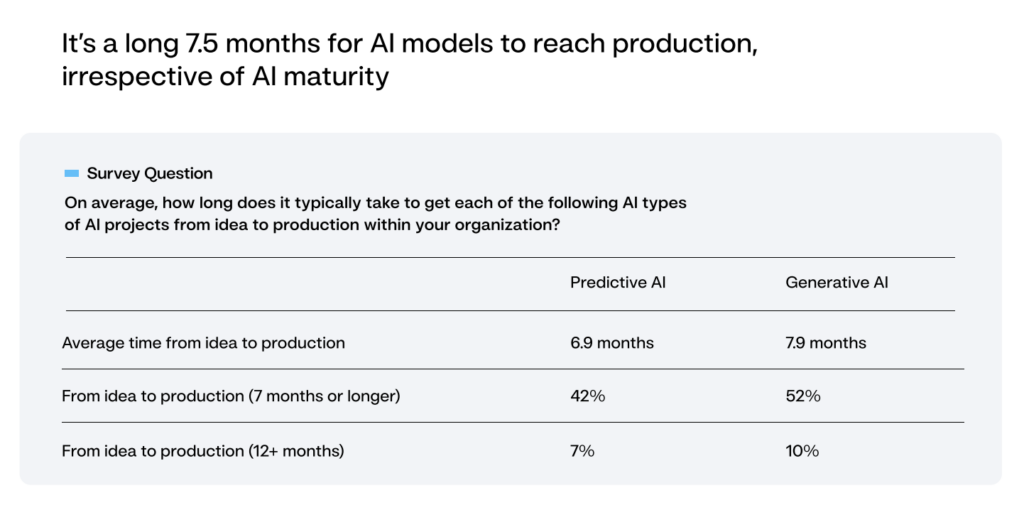
To safeguard mannequin integrity, AI leaders ought to:
- Automate documentation, versioning, and historical past monitoring.
- Put money into applied sciences with customizable guards and deep observability at each step.
- Empower AI groups to simply and constantly check, validate, and examine fashions.
- Present collaborative workspaces and centralized hubs for seamless communication and handoffs.
- Set up well-monitored information pipelines to forestall drift, and keep information high quality and consistency.
- Emphasize the significance of mannequin documentation and conduct common audits to satisfy compliance requirements.
- Set up clear standards for when to replace or keep fashions, and develop a rollback technique to shortly revert to earlier variations if wanted.
By adopting these practices, AI leaders can guarantee excessive requirements of mannequin integrity, cut back threat, and ship impactful outcomes.
Prepared the ground in AI collaboration and innovation
As an AI chief, you might have the facility to create environments the place collaboration and innovation thrive.
By selling shared information, clear communication, and collective problem-solving, you may maintain your groups motivated and targeted on high-impact outcomes.
For deeper insights and actionable steerage, discover our Unmet AI Wants report, and uncover find out how to strengthen your AI technique and crew efficiency.
In regards to the writer
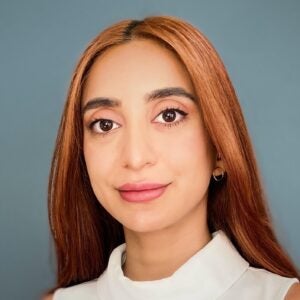
Could Masoud is an information scientist, AI advocate, and thought chief educated in classical Statistics and trendy Machine Studying. At DataRobot she designs market technique for the DataRobot AI Governance product, serving to world organizations derive measurable return on AI investments whereas sustaining enterprise governance and ethics.
Could developed her technical basis by levels in Statistics and Economics, adopted by a Grasp of Enterprise Analytics from the Schulich College of Enterprise. This cocktail of technical and enterprise experience has formed Could as an AI practitioner and a thought chief. Could delivers Moral AI and Democratizing AI keynotes and workshops for enterprise and educational communities.